Redenbacher POC
Overview
Popcorn (details below) is a new app concept from WarnerMedia that is being tested in Q2 2020. The current version involves humans curating “channels” of content, which is sourced through a combination of automated services, crowdsourcing, and editorially selected clips. Project Redenbacher takes the basic Popcorn concept, but fully automates the content/channel selection process through an AI engine.
Popcorn Overview
Popcorn is a new experience, most easily described as “TikTok meets HBOMax”. WarnerMedia premium content is “microsliced” into small clips (“kernels”), and organized into classes (“channels”). Users can easily browse through channels by swiping left/right, and watch and browse a channel by swiping up/down. Kernels are short (under a minute), and can be Favorited or Shared directly from the app. Channels are organized thematically, for example “Cool Car Chases” or “Huge Explosions” or “Underwater Action Scenes”. Channels comprise content generated by the HBO editorial team, popularity/most watched, and from AI/algorithms.
Demo
removed
Redenbacher Variant
Basic Logic
In Redenbacher, instead of selecting a specific channel, the user starts with a random clip. The AI selects a Tag that’s associated with the clip, and automatically queues up a next piece of content that has that same Tag in common. This continues until the user interacts with the stream.
Possible actions (built on same logic/flow as per Popcorn):
- Swipe Up: go to previous clip
- Swipe Down: go to next clip (using same rules)
- Swipe Left/Right: play another clip that contains the same Tag as the one currently playing (aka “swap out” this clip for another)
- Restart: restarts the entire sequence from another random clip
ContentAI
ContentAI provides the ability to use the latest and greatest computer vision and NLP models provided by cloud providers AWS, Azure and GCP as well as custom models contributed to the platform from our partners, AT&T Research Labs and Xandr.
Data
Discovery
The data that was extracted for the SceneFinder POC was also used for the Redenbacher POC. We grouped tags up to the second. Each extractor provides varying levels of frames analyzed per second. Rolling the tags up to the nearest second made it easy to develop against without data loss.
Loader
Redenbacher is an extension of the extracted metadata and learnings from the SceneFinder POC.
We identified where tags were present on the screen for longer than five seconds. Then created a video clip for that tag. We also saved the other tags identified in the clip to be used within the app.
Let's look at a simple example
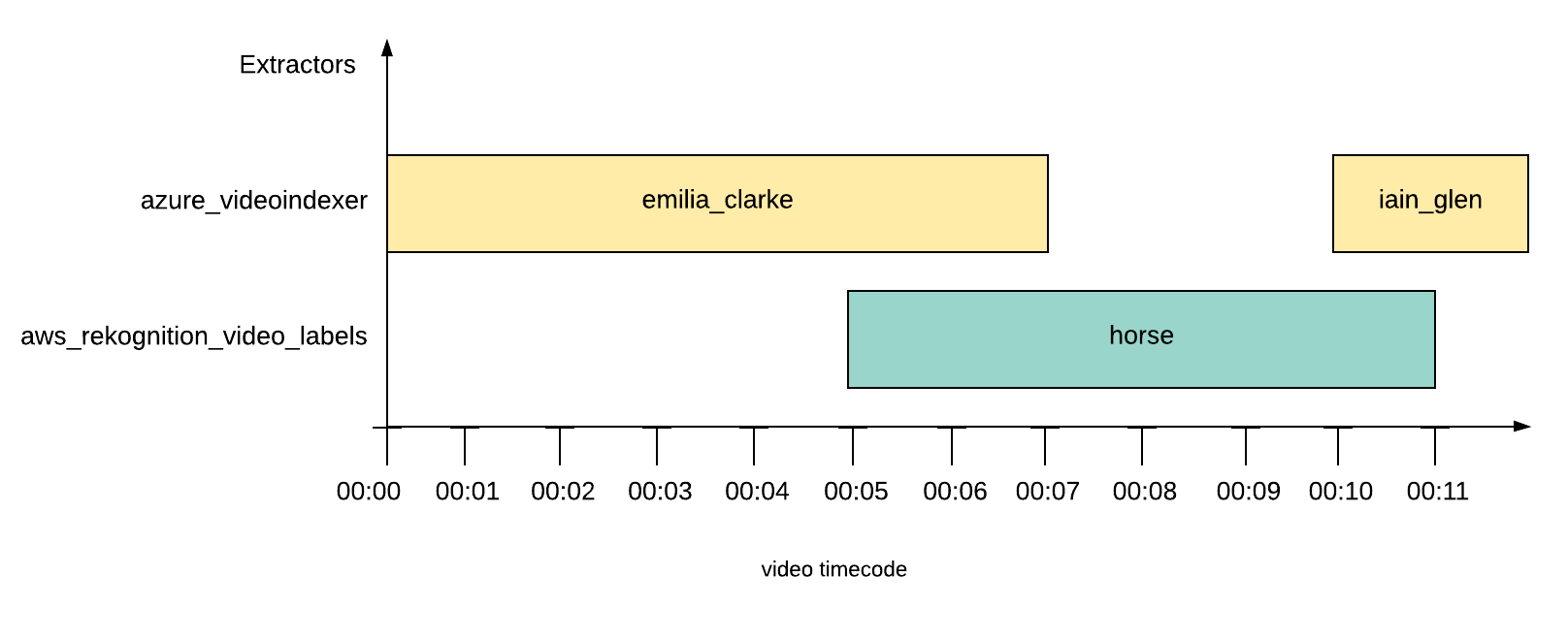
We will create videos for tags
- emilia_clarke from 00:00 to 00:07
- horse from 00:05 to 00:11
*We will not create a video clip for iain_glen since he was only on the screen for two seconds.
If tags from other extractors exist within the video we created, those tags we be included in the JSON output within an tags collection
- emilia_clarke - includes horse
- horse - includes emilia_clarke and iain_glen
Sample output
[
{
"name": "emilia_clarke",
"clips": [
{
"franchise": "Game of Thrones",
"title": "winter is coming",
"season": 1,
"episode": 1,
"key": "videos/popcorn/emilia_clarke/ai_got_01_winter_is_coming_263255_PRO35_10-out_000000_000007.mp4",
"tags": [
"horse"
]
}
],
},
{
"name": "horse",
"clips": [
{
"franchise": "Game of Thrones",
"title": "winter is coming",
"season": 1,
"episode": 1,
"key": "videos/popcorn/horse/ai_got_01_winter_is_coming_263255_PRO35_10-out_000005_000011.mp4",
"tags": [
"emilia_clarke",
"iain_glen"
]
}
]
}
]
Video clip creation
We leveraged AWS MediaConvert to create the video clips.
Video clip creation fun facts
- Produced ~4,300 video clips
- Processing took ~20 minutes
- Average video length 00:35
- 190 unique tags
Website
Functionality
The app contains clips from the first season of Game of Thrones. On the start of the app, you will receive a random video from the collection of clips we created. We will save the tag
associated with the video and allow you to perform a few actions
- Show a different
tag
scene- return a video clip we haven't shown in this session with the same
tag
- return a video clip we haven't shown in this session with the same
- Back to
tag
- navigate back to the previous video(s) played
- Next clip
- we will select a random
tag
from thetags
collection to act as the maintag
moving forward
- we will select a random
- Show a random clip
- start over
The website will display the tag
(bold), and all tags
associated with the clip
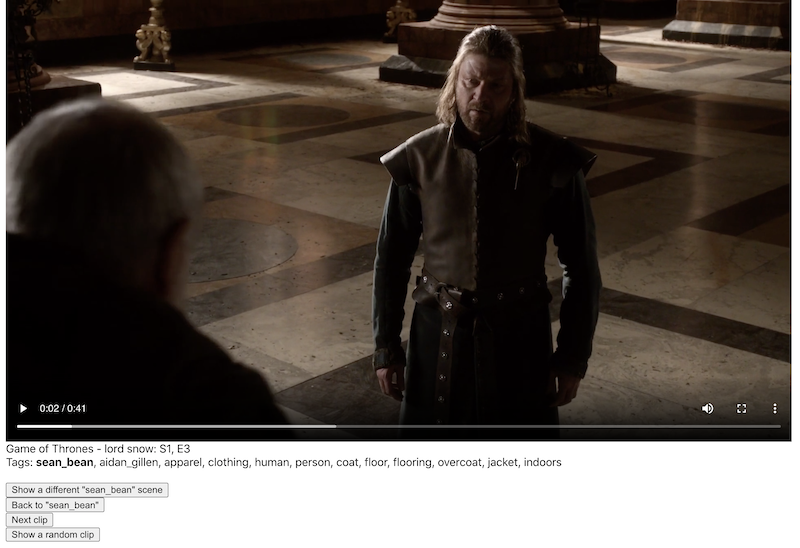
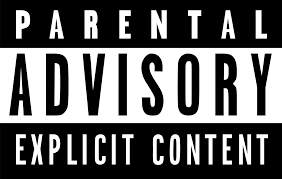
Summary
Users love consuming short-form videos. In this blog we demonstrated using the simplicity of ContentAI to call AI Services from Microsoft, AWS and Google. As well as use the scale of AWS Media Convert to create thousands of short-form video clips. The Redenbacher app is intended to be a fun way to quickly navigate through the content. Give it a shot, we hope you like it.
Future Enhancements
- AT&T Research and Xandr have done some amazing work training custom models for the first iteration of Popcorn. We will continue our relationship and keep adding new extractors with their new pertained models to run inference across any video ContentAI can access.
- Human in the loop for clip validation and modification if needed.
- For this POC the script to create videos using AWS Media Convert was run from a local machine, in the future we will incapsulate this functionality into an extractor that runs on the platform.
Cost
We ran extractors and created clips for all 10 episodes for the first season of Game of Thrones. Roughly 10 hours of content
ContentAI
Extractor | Cost |
---|---|
gcp_videointelligence_label | $60.59 |
gcp_videointelligence_logo_recognition | $90.59 |
azure_videoindexer | $114.08 |
aws_rekognition_video_labels | $60.08 |
Total | $325.34 |
One of the many benefits of ContentAI is this is a one time cost. Now that the results are stored in our Data Lake they can be used by any application in the future.
To learn more about calculating the cost for your project please see visit our cost calculator page. Also, if you would like to learn more about getting the data from our Data Lake to use in your application, please check out our CLI, HTTP API and/or GraphQL API docs.
Contributors
- Jeremy Toeman - WarnerMedia Innovation Lab
- Scott Havird - WarnerMedia Cloud Platforms
Related Work
Dunk Detector
Uses GCP Video Intelligence Label extractor. The result set provides a dunk
tag with start and end times for when the action occurred on the screen. Instead of creating clips for each segment, for this showcase app we simply load the time segments into a playlist.
AWS Rekognition Video Content Moderation
Uses AWS Rekognition Video Content Moderation extractor. Instead of creating clips for each segment, for this showcase app we simply load the time segments into a playlist when you click on a label. You also have the option to play an individual video segment by simply clicking on it.